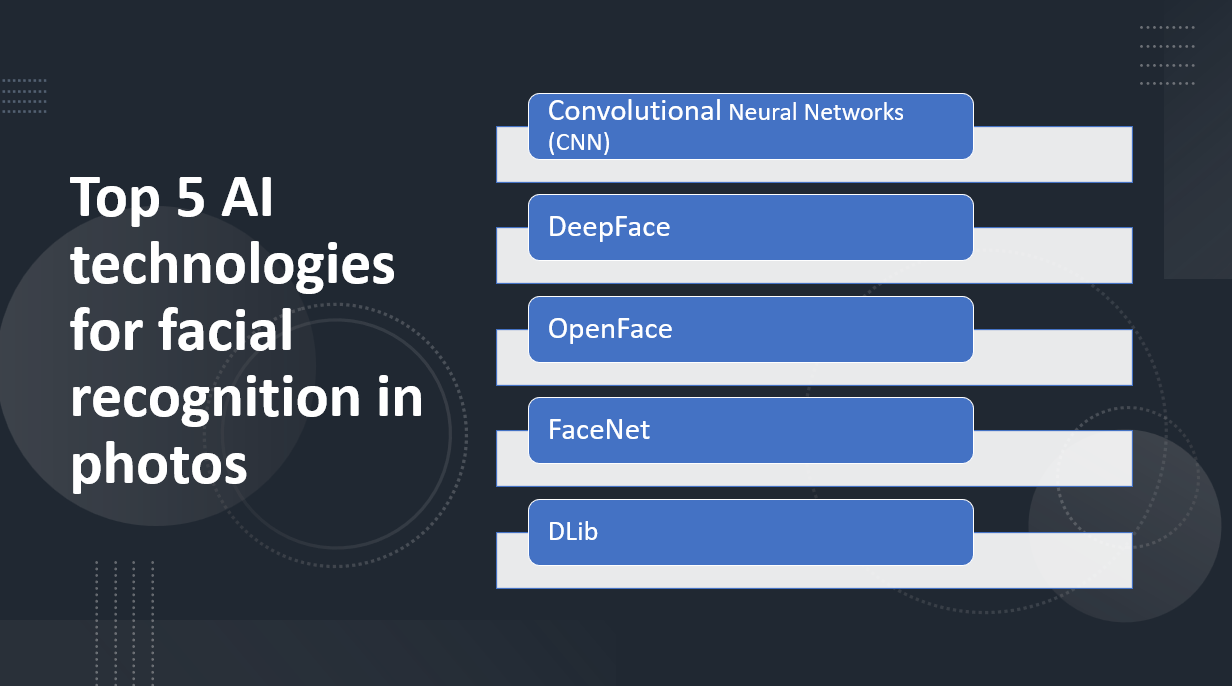
Introduction
Facial recognition technology has seen significant advancements in recent years, revolutionizing various industries such as security, surveillance, and personal identification.
Artificial Intelligence (AI) plays a crucial role in powering facial recognition systems, enabling accurate and efficient identification of individuals in photos.
In this blog post, we will explore the top five AI technologies for facial recognition in photos and their contributions to enhancing security and streamlining identification processes.
Why use AI technologies for facial recognition in photos
- AI technologies provide precise facial recognition in photos.
- AI systems automate the process, enabling fast analysis of large photo collections.
- AI can handle large-scale datasets, suitable for diverse applications.
- AI can recognize faces across various conditions, angles, and expressions.
- Facial recognition in photos improves access control and identity verification.
Here Are Our Top 5 AI technologies for facial recognition in photos:
1: Convolutional Neural Networks (CNN)
Overview and Importance
Convolutional Neural Networks (CNN) are a fundamental AI technology extensively used for facial recognition in photos. These networks have transformed the field of computer vision, allowing machines to automatically extract features and recognize intricate patterns in images, particularly focusing on facial attributes.
Learn more about Convolutional Neural Networks (CNN)
Key Techniques and Approaches
CNN Architectures
- Utilize popular CNN architectures like VGG, ResNet, and Inception known for their depth and ability to learn hierarchical representations of facial features.
Face Detection, Alignment, and Recognition
- Incorporate techniques such as face detection to locate faces in an image, face alignment to normalize facial pose and position, and face recognition to match faces to known identities, forming integral components of CNN-based facial recognition systems.
2: DeepFace
Overview and Importance
DeepFace is an AI technology developed by Facebook's Artificial Intelligence Research (FAIR) team, specifically designed for facial recognition tasks. It gained significant attention and importance due to its remarkable accuracy and capability to recognize faces with high precision.
Learn more about DeepFace
Key Features and Capabilities
DeepFace utilizes a deep convolutional neural network architecture that processes facial images through multiple layers to extract meaningful features.
Some key features and capabilities of DeepFace include:
Face Verification
- DeepFace can determine whether two facial images belong to the same person or not, enabling identity verification.
Facial Landmark Detection
- It can accurately detect and locate key facial landmarks such as eyes, nose, and mouth, which aids in face alignment and analysis.
Pose-Invariant Representation
- DeepFace's architecture is designed to generate pose-invariant facial representations, allowing it to recognize faces across different head poses.
Robustness to Variations
- DeepFace is designed to handle variations in lighting conditions, facial expressions, and partial occlusions, making it more robust in real-world scenarios.
3: OpenFace
Overview and Importance
OpenFace is an open-source facial recognition framework developed by the Computer Vision and Machine Learning Group at Carnegie Mellon University. It plays a significant role in advancing facial recognition technology and promoting research and development in the field.
Learn more about OpenFace
Key Features and Capabilities
OpenFace offers a range of key features and capabilities that contribute to its effectiveness in facial recognition:
Face Embeddings
- OpenFace can extract high-dimensional embeddings from facial images, representing unique characteristics of each individual's face.
Facial Landmark Detection
- It includes a robust facial landmark detection module that accurately identifies key facial points, aiding in alignment and analysis.
Deep Neural Networks
- OpenFace employs deep neural networks, specifically deep convolutional neural networks (CNNs), to learn discriminative features and patterns from facial data.
Real-time Processing
- OpenFace is designed to process facial images in real-time, making it suitable for applications requiring fast and efficient face recognition.
4: FaceNet
Overview and Importance
FaceNet is a deep learning model for facial recognition developed by researchers at Google. It has gained significant importance in the field of computer vision and face recognition due to its innovative approach and high accuracy in identifying and verifying faces.
Learn more about FaceNet
Key Features and Capabilities
FaceNet offers several key features and capabilities that contribute to its effectiveness in facial recognition:
Triplet Loss
- FaceNet utilizes a triplet loss function that learns a feature representation for each face, ensuring that the embeddings of the same person's face are closer to each other than those of different individuals.
Deep Convolutional Neural Networks (CNNs)
- FaceNet employs deep CNNs to extract facial features, encoding them into compact and discriminative representations.
Face Embeddings
- The model generates a fixed-length numerical embedding for each face, capturing its unique characteristics in a high-dimensional space.
Face Verification and Identification
- FaceNet enables both face verification, where it determines whether two faces belong to the same person, and face identification, where it assigns an identity to a given face by comparing it with a database of known faces.
5: DLib
Overview and Importance
DLib is a popular open-source library for machine learning and computer vision that includes robust tools and algorithms for facial recognition. It is widely used in both academic research and industry applications, making it an important resource in the field of facial recognition.
Learn more about DLib
Key Features and Capabilities
DLib offers several key features and capabilities that contribute to its effectiveness in facial recognition:
Face Detection
- DLib provides highly accurate face detection algorithms that can locate and localize faces within an image or video stream.
Facial Landmark Detection
- It includes algorithms to detect facial landmarks, such as the positions of the eyes, nose, and mouth. This information can be used for face alignment and pose estimation.
Face Embeddings
- DLib can generate high-dimensional face embeddings that capture the unique features of each face. These embeddings can be used for face verification, identification, and clustering.
Face Recognition
- DLib implements robust face recognition algorithms that can match and identify faces from a database of known individuals.
Pre-Trained Models
- DLib provides pre-trained models for face detection, facial landmark detection, and face recognition, making it easier to get started with facial analysis tasks.
Conclusion
Facial recognition technology has significant importance in various applications, ranging from security and identification processes to personalized experiences. AI technologies play a crucial role in advancing facial recognition capabilities, enabling accurate and efficient analysis of facial features in photos.
The top five AI technologies for facial recognition in photos are:
Convolutional Neural Networks (CNN): CNNs excel in feature extraction and pattern recognition, making them effective for facial analysis tasks. They can detect faces, align facial landmarks, and recognize individuals with high accuracy.
DeepFace: DeepFace is a specialized facial recognition system developed by Facebook. It utilizes deep learning techniques to analyze facial features and achieve remarkable accuracy in face matching and identification.
OpenFace: OpenFace is an open-source library that provides comprehensive facial analysis tools. It offers face detection, landmark detection, and face recognition capabilities, allowing for robust and customizable facial recognition solutions.
FaceNet: FaceNet is a deep learning model that generates facial embeddings, representing unique features of each face. It enables accurate face matching and verification, even across different images and poses.
DLib: DLib is an open-source library that offers facial detection, landmark detection, and face recognition capabilities. It provides high accuracy, efficiency, and flexibility, making it a popular choice for facial recognition tasks.
AI technologies for facial recognition have key features like face detection, landmark identification, accurate matching, and personalized experiences. They improve security, streamline identification processes, and revolutionize various industries. Responsible use, privacy protection, and addressing biases and limitations are crucial considerations. CNN, DeepFace, OpenFace, FaceNet, and DLib are five top AI technologies driving innovation in facial recognition, delivering accurate analysis and identification while respecting privacy and consent. Embracing ethical practices ensures continued progress in this field.