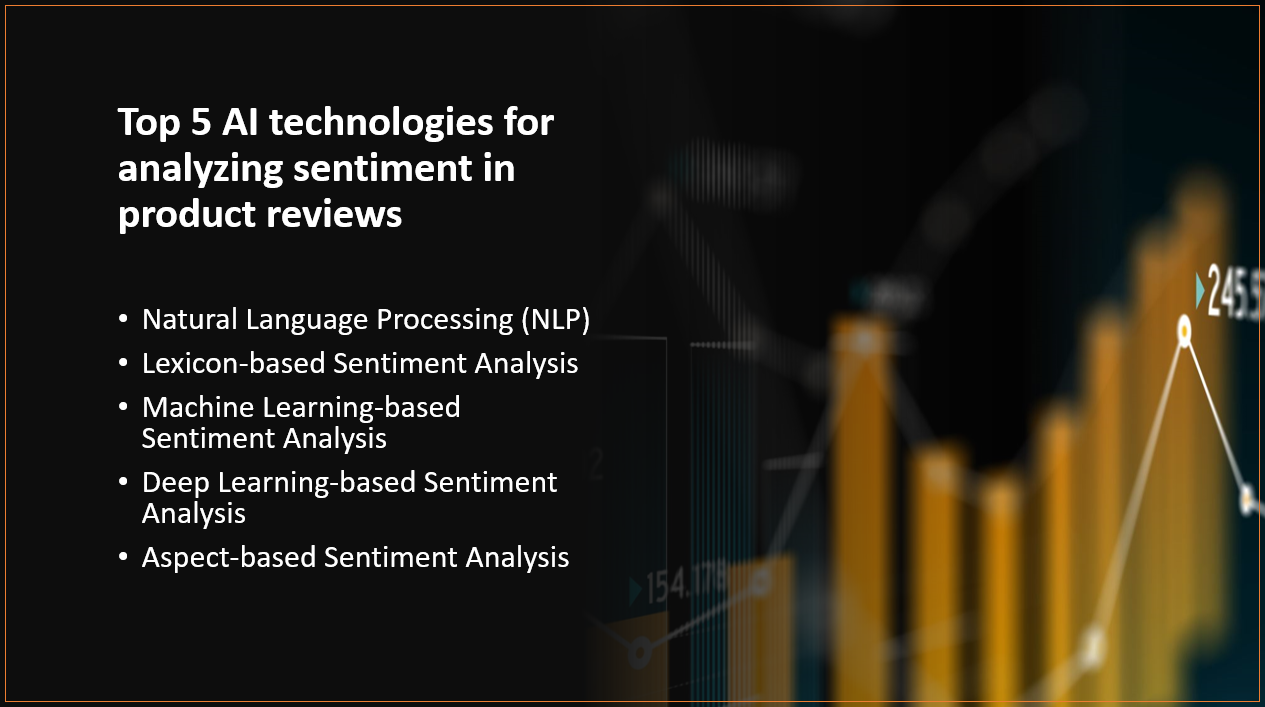
Introduction
Analyzing sentiment in product reviews is crucial for businesses to understand customer opinions, make data-driven decisions, and improve their products and services. Artificial Intelligence (AI) technologies have revolutionized sentiment analysis by automating the process of extracting sentiments and opinions from textual data. In this blog post, we will explore the top five AI technologies for analyzing sentiment in product reviews, empowering businesses to gain valuable insights and enhance customer experiences.
Why use AI Technologies for Analyzing Sentiment in Product Reviews?
- AI technologies provide precise sentiment analysis of product reviews.
- AI automates the analysis process, saving time and effort.
- AI-powered sentiment analysis offers valuable insights for product improvements and marketing strategies.
- AI enables real-time monitoring and response to customer sentiment.
Here Are Our Top 5 AI technologies for analyzing sentiment in product reviews:
1: Natural Language Processing (NLP)
Overview and Importance
Natural Language Processing (NLP) is an AI technology that focuses on the interaction between computers and human language. NLP plays a crucial role in extracting meaningful insights and understanding the sentiment expressed by customers. By leveraging various NLP techniques and algorithms, businesses can gain valuable insights into customer opinions, preferences, and satisfaction levels.
Key Features and Capabilities
Text Preprocessing
- NLP techniques are used to preprocess and clean textual data, including tasks such as tokenization, stop word removal, and stemming, which enhance the accuracy of sentiment analysis.
Sentiment Analysis
- NLP algorithms enable the identification and classification of sentiment polarity in product reviews, distinguishing between positive, negative, and neutral sentiments.
Aspect-Based Sentiment Analysis
- NLP models can analyze specific aspects or features of a product mentioned in reviews and determine the sentiment associated with each aspect.
2: Lexicon-based Sentiment Analysis
Overview and Importance
Lexicon-based sentiment analysis is an AI technology that relies on predefined sentiment lexicons or dictionaries to analyze the sentiment expressed in text. lexicon-based approaches are valuable as they provide a quick and efficient way to determine sentiment polarity without requiring extensive training data. Lexicon-based sentiment analysis is particularly useful for domains where domain-specific sentiment lexicons are available, enabling businesses to assess customer sentiment accurately.
Key Features and Capabilities
Sentiment Lexicons
- Lexicon-based sentiment analysis utilizes prebuilt sentiment lexicons containing words and their associated sentiment polarities (positive, negative, or neutral).
Polarity Scoring
- Sentiment analysis algorithms assign sentiment scores to text by calculating the cumulative sentiment polarity of words present in the lexicon.
Contextual Analysis
- Lexicon-based approaches can account for contextual information, such as negation words or intensifiers, to refine the sentiment analysis results.
3: Machine Learning-based Sentiment Analysis
Overview and Importance
Machine Learning-based sentiment analysis is an AI technology that utilizes machine learning algorithms to automatically classify the sentiment expressed in product reviews. By training models on labeled datasets, machine learning algorithms learn patterns and relationships between text features and sentiment labels, enabling accurate sentiment analysis. Machine Learning-based approaches are crucial for analyzing sentiment in product reviews as they can handle complex language nuances and adapt to different domains.
Key Features and Capabilities
Training on Labeled Data
- Machine learning models for sentiment analysis are trained on labeled datasets where each review is annotated with its corresponding sentiment label (positive, negative, or neutral).
- Machine learning algorithms extract relevant features from the text, such as n-grams, word embeddings, or syntactic structures, to capture sentiment-related information.
Classification Algorithms
- Machine learning models use various classification algorithms, such as Support Vector Machines (SVM), Naive Bayes, or Neural Networks, to classify reviews into sentiment categories.
4: Deep Learning-based Sentiment Analysis
Overview and Importance
Deep Learning-based sentiment analysis is an AI technology that leverages deep neural networks to automatically analyze and classify sentiment in product reviews. By utilizing complex architectures and large-scale training on labeled datasets, deep learning models can capture intricate patterns and dependencies in text, leading to highly accurate sentiment analysis. Deep learning has revolutionized sentiment analysis by providing advanced techniques to handle the nuances of language and extract nuanced sentiment information from product reviews.
Key Features and Capabilities
Neural Network Architectures
- Deep learning models for sentiment analysis employ various architectures, such as Recurrent Neural Networks (RNNs), Convolutional Neural Networks (CNNs), or Transformer models, to capture contextual information and extract sentiment features.
Word Embeddings
- Deep learning models utilize word embeddings, such as Word2Vec or GloVe, to represent words as dense vectors, capturing semantic relationships and contextual meaning.
Transfer Learning
- Deep learning models can benefit from transfer learning by leveraging pre-trained models on large corpora, enabling better sentiment analysis performance even with limited labeled data.
5: Aspect-based Sentiment Analysis
Overview and Importance
Aspect-based sentiment analysis is an AI technology that focuses on analyzing the sentiment expressed towards specific aspects or features of a product or service mentioned in reviews. It aims to provide a more fine-grained understanding of sentiment by considering the different aspects that customers discuss. By identifying and analyzing the sentiment associated with each aspect, businesses can gain deeper insights into customer opinions, strengths, and weaknesses of their products, and make informed decisions for improvement.
Key Features and Capabilities
- Aspect-based sentiment analysis involves automatically identifying and extracting the aspects or features mentioned in product reviews. This can be achieved through techniques like rule-based extraction or machine learning-based approaches.
Sentiment Classification
- Once the aspects are identified, sentiment classification techniques are employed to determine the sentiment polarity (positive, negative, or neutral) associated with each aspect. This can be done using techniques like lexicon-based methods or machine learning algorithms.
Fine-grained Analysis
- Aspect-based sentiment analysis enables a more granular analysis by associating sentiment with specific aspects, allowing businesses to pinpoint areas of improvement or areas of strength.
Conclusion
AI technologies play a significant role in analyzing sentiment in product reviews, enabling businesses to extract valuable insights from customer feedback and make data-driven decisions. The top five AI technologies for sentiment analysis are Natural Language Processing (NLP), Lexicon-based Sentiment Analysis, Machine Learning-based Sentiment Analysis, Deep Learning-based Sentiment Analysis, and Aspect-based Sentiment Analysis.
Here are their key features, capabilities, and advantages:
Natural Language Processing (NLP): Utilizes linguistic rules and statistical models to analyze text and extract sentiment from product reviews.
Lexicon-based Sentiment Analysis: Relies on predefined sentiment lexicons to assign sentiment scores to words and phrases, allowing for quick and interpretable sentiment analysis.
Machine Learning-based Sentiment Analysis: Trains models on labeled data to classify sentiments in text, providing more accurate sentiment analysis results.
Deep Learning-based Sentiment Analysis: Utilizes deep neural networks to learn complex patterns and representations in text, achieving high accuracy in sentiment classification.
Aspect-based Sentiment Analysis: Focuses on identifying sentiments towards specific aspects or features of products, providing a more granular understanding of customer feedback.
Sentiment analysis has a significant impact on businesses:
Understanding customer feedback: Sentiment analysis allows businesses to gauge customer satisfaction, identify areas for improvement, and address concerns proactively.
Product improvement: Insights from sentiment analysis can drive product enhancements, enabling businesses to meet customer expectations and preferences.
Customer satisfaction: By analyzing sentiment in product reviews, businesses can take action to enhance customer satisfaction, leading to improved loyalty and retention.
Businesses are encouraged to leverage these AI technologies and sentiment analysis techniques. By applying sentiment analysis to product reviews, businesses can gain valuable insights, make data-driven decisions, and continuously improve their products and services. Sentiment analysis empowers businesses to be more customer-centric, enhancing customer satisfaction and driving business success.